I love using Strava to track my bike rides, it's light on the battery, and there's even a handy little homescreen widget I can use to start up a new ride. Now on top of that, I found out that they also let you download all your activity history, which is great because I've been wanting to familiarize myself a little bit with Jupyter/Pandas/Matplotlib lately. It's actually pretty neat, and you can get some interesting results pretty quickly.
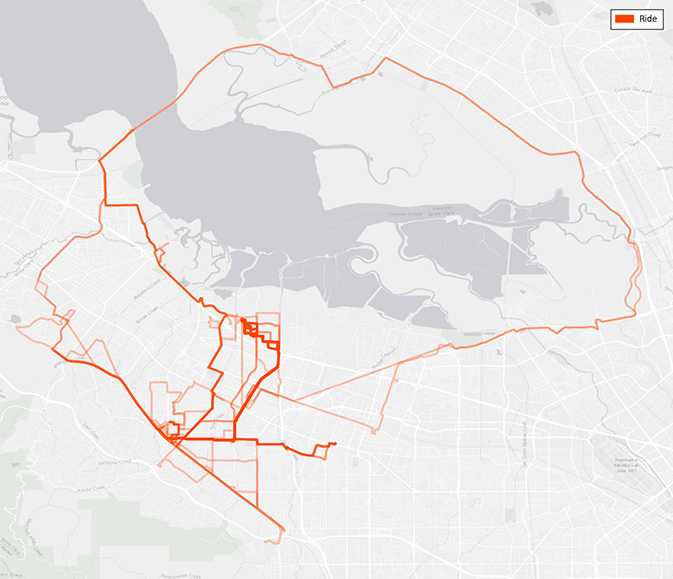
A heat map of my tracked bike rides around the Bay Area. The loop around the bay is a good ride, except for a small patch just past the Dumbarton Bridge where it is not paved.
The dump from Strava is provided as a zip of GPX files, one for each tracked activity including metadata and raw waypoint data (lat/long, elevation, time, etc). The waypoint data is suprisingly precise and abundant, I had over 560k waypoints for the only 300+ rides since I started tracking, and from that you can pretty easily calculate the basic Strava information from their site; distance of each ride (using the Haversine formula as I found out), average speed, elevation climb, and moving time for example.
In my case, I wanted more information about my commute, so I broke down the rides into the three categories; morning, evening and leisure, and by joining that with some weather data from Darksky, I found some pretty interesting things about my rides.
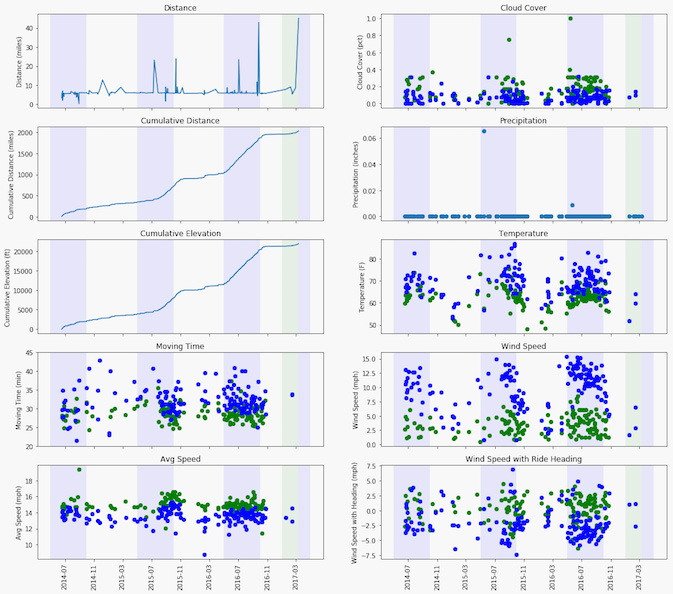
Some descriptive statistics of my riding data. The wind speed with heading was approximated by taking the dot product of the wind vector (at the ride start) and the normalized ride vector scaled by the wind speed.
Each point in the plots is a ride; green for a morning commute and blue for an evening commute. The blue backgrounds indicate "summer" months, and the green-ish background indicates the current year. From a first glance, you can see that I don't really ride in the rain (hence no precipitation data :), and the majority of my tracked rides are accounted for by commutes to and from work. If you add up the cumulative elevation change, I've also ridden higher than Mt. Fuji now, which is pretty cool!
Looking at the rest of the data, I can also find things that correlate with my experience riding over the last couple years. It's clear that I am about 2mph (2-3min) faster riding to work than from it. I've always blamed it on end-of-day tiredness and the elevation change getting back up the hills towards the Santa Cruz mountains, but it looks like there are an additional environmental effects. The increase in average temperature (10°F) doesn't help, nor does wind from the Pacific in the early evening blowing opposite to my riding direction, which I had never thought much about until now looking at the graph.
I have some more ideas for how to play with the data, but now I really wish Strava had been around back in 2007-2010 when I was riding my bike to downtown Toronto for work – that data would have been really interesting to see!